Massive MIMO Systems
Staff Members of the Project
- Prof. Dr.-Ing. Robert Schober
- Prof. Dr.-Ing. Wolfgang Gerstacker
- Shahram Zarei, M.Sc.
- Yongpeng Wu, Ph.D.
- Jiayi Zhang, Ph.D.
1. Introduction
With the increasing demand for higher data rates, multiple-input multiple-output (MIMO) systems have attracted much attention over the last decade [1]. Today, MIMO technology is a key element of many modern wireless communication standards including Long Term Evolution (LTE) and WiMAX. In contrast to single-input single-output (SISO) systems, where both transmitters and receivers are equipped with a single antenna, in MIMO communication systems, transmitters and/or receivers employ several antennas. It is well known that MIMO communication systems achieve substantial gains in spectral, power, and energy efficiency compared to conventional single-input single-output (SISO) systems [1]. In fact, it has been shown that under ideal conditions the capacity of a point-to-point MIMO system with NTNT transmit antennas and NRNR receive antennas scales linearly with min{NTNT, NRNR}, which is referred to as the multiplexing gain in the literature [2, 3]. However, point-to-point MIMO systems have several disadvantages in practice. First, the number of antennas that a mobile terminal (e.g., a smart phone) can accommodate is constrained due to size, power consumption, and cost constraints, which limits the multiplexing gain. Second, the multiplexing gain may disappear altogether in case of strong interference (e.g., at the cell edges), unfavorable channel conditions (e.g., insufficient scattering), and narrow antenna spacing mandated by the size constraints of mobile terminals. The disadvantages of point-to-point MIMO systems can be overcome by multi-user MIMO systems [4, 5, 6]. In multi-user MIMO systems, a central node with multiple antennas (e.g., a base station) serves a number of (mobile) users with a small number of antennas. Thus, the signal processing complexity at the mobile terminals is low, especially in case of single-antenna terminals. In addition, since the users are spatially distributed over an entire cell, the angular separation of the terminals typically exceeds the Rayleigh resolution of the array and the channels of different users can be assumed independent. However, the multiple users in the system introduce inter-user interference which has to be mitigated by appropriate processing at the transmitter and receiver for downlink (i.e., base station to users) and uplink (i.e., users to base station) transmission, respectively. However, point-to-point MIMO systems have several disadvantages in practice. First, the number of antennas that a mobile terminal (e.g., a smart phone) can accommodate is constrained due to size, power consumption, and cost constraints, which limits the multiplexing gain. Second, the multiplexing gain may disappear altogether in case of strong interference (e.g., at the cell edges), unfavorable channel conditions (e.g., insufficient scattering), and narrow antenna spacing mandated by the size constraints of mobile terminals. The disadvantages of point-to-point MIMO systems can be overcome by multi-user MIMO systems [4, 5, 6]. In multi-user MIMO systems, a central node with multiple antennas (e.g., a base station) serves a number of (mobile) users with a small number of antennas. Thus, the signal processing complexity at the mobile terminals is low, especially in case of single-antenna terminals. In addition, since the users are spatially distributed over an entire cell, the angular separation of the terminals typically exceeds the Rayleigh resolution of the array and the channels of different users can be assumed independent. However, the multiple users in the system introduce inter-user interference which has to be mitigated by appropriate processing at the transmitter and receiver for downlink (i.e., base station to users) and uplink (i.e., users to base station) transmission, respectively.
An emerging research area in multi-user MIMO communications are so-called massive MIMO or large-scale MIMO systems, where in contrast to conventional multi-user MIMO systems, which employ about ten antennas at the base station, base stations are equipped with much larger numbers of antennas, e.g., one hundred or more [7]. In Fig. 1, a downlink massive MIMO system is shown. Although such a tremendous increase in the number of antennas introduces new challenges for transceiver design and implementation, it has some interesting advantages for signal processing and communication [8]. For example, if the number of base station antennas is much larger than the number of users in the system, simple conjugate beamforming (BF) precoding (downlink) and matched filter (MF) detection (uplink) at the base station lead to close-to-optimal performance facilitating low complexity signal processing at both the base station and the user terminals [9]. Furthermore, random impairments such as small-scale fading and noise are averaged out as the number of base station antennas grows large [9]. To keep the signaling overhead for channel state information (CSI) acquisition in massive MIMO systems manageable, time division duplex (TDD) operation is preferred, since for frequency division duplex (FDD) systems the amount of CSI feedback grows with the number of base station antennas. A major impairment in massive MIMO systems is the so-called pilot contamination. Pilot contamination is caused by the reuse of the same (or linearly dependent) pilot sequences in different cells. This reuse is unavoidable as, for a given pilot sequence length, the number of linearly independent pilot sequences is limited. However, recently several efficient techniques have been proposed to overcome pilot contamination [10, 11]. Although massive MIMO communication systems have been first proposed in 2010 [7], they have attracted considerable interest from both academia and industry in a very short time. For example, a 2013 special issue of the IEEE Journal on Selected Areas in Communications was dedicated solely to massive MIMO systems and massive MIMO plays an important role in GreenTouch’s quest to improve the energy efficiency of communication networks by 2015 by a factor of 1000 compared to the 2010 levels. GreenTouch is an industry consortium comprising many major companies such as Bell Labs, Broadcom Corporation, China Mobile, Huawei, Swisscom, and Vodafone, see http://www.greentouch.org. In fact, it has been shown in [9] that if NT grows large and all other system parameters are assumed constant, the transmit power per user in multi-user massive MIMO systems can be reduced proportionally to 1/NT1/NT and 1/NT−−−√1/NT for perfect and imperfect CSI knowledge at the base station, respectively, without affecting throughput and reliability. Hence, massive MIMO systems offer a simple path to more energy efficient and „greener“ communication networks. Considering the favorable properties of and large interest in massive MIMO systems, we expect them to become an integral part of future wireless communication systems. Although a considerable research effort has already been directed towards massive MIMO systems, several important signal processing and signal design problems remain to be solved. In the next section, some of the massive MIMO research topics that IDC is working on are presented.

2. Low-Complexity Linear Precoding
Although BF precoding has a low complexity and achieves a high performance as the number of antennas goes to infinity for a fixed number of users, in practice, the maximum number of antennas will be limited and the number of users in a cell may be large [9]. For this scenario, it has been shown that the MMSE precoder achieves a considerable performance gain compared to the BF precoder [12, 13]. The MMSEprecoding matrix is given by
VMMSE=ξMMSEHH(HHH+σ2nIK)−1,
where HH, σ2nσn2, and IKIK denote the channel matrix, the noise variance, and the K×KK×K identity matrix, respectively. ξMMSEξMMSE is a normalization factor, which ensures that the transmit power constraint tr(VMMSEVHMMSE)tr(VMMSEVMMSEH)=PTXPTX is met, where PTXPTX is the total transmit power at the base station. The MMSE precoder still entails a high complexity due to the required matrix inversion, especially for the large matrices typical for massive MIMO systems. To overcome this problem, in our recent work, we approximated the matrix inversion in the MMSE precoder by a matrix polynomial, which leads to the following polynomial expansion (PE) precoder matrix [15]
VPE=ξPEHH∑Ll=0ωl(HHH)l,VPE=ξPEHH∑l=0Lωl(HHH)l,
where ξPEξPE is a normalization factor, which ensures that the transmit power constraint tr(VPEVHPE)tr(VPEVPEH)=PTXPTX is fulfilled and the coefficients ωlωl in VPEVPE can be determined by solving an optimization problem, e.g., for sum-MSE minimization or sum rate maximization. Moreover, in our recent work [15], we have shown that the optimal coefficients ωlωl do not depend on the instantaneous channel realizations and can be determined easily using tools from random matrix theory. Furthermore, we have shown that for large matrix polynomial orders LL, the proposed PE precoder approaches the sum rate of the MMSE precoder. The sum rate performance of the PE precoder for a system with N=100N=100 base station antennas is depicted in Fig. 2. As can be seen, if the number of users is small compared to the number of antennas, all precoding schemes achieve almost the same sum rate which confirms that in such scenarios BF precoding is preferable, since it requires a lower computational complexity than all other schemes. With increasing number of users, the performance gap between the proposed PE precoding scheme and the BF precoding scheme increases and has a peak at K=NK=N. From Fig. 2, it can also be concluded that for K/N=1K/N=1, even for L=1L=1 a sum rate improvement of more than 40%40% compared to BF precoding can be achieved with the proposed PE precoder. For the same K/NK/N and L=2L=2, the proposed PE precoder achieves more than 86%86% of the sum rate of the MMSE precoder.

Research Challenges and Open Problems
Some open issues in design of PE precoders include:
- Consideration of multi-cell interference and pilot contamination
The PE precoder in [15] has been designed for a single-cell scenario. However, multi-cell networks are more realistic application scenarios and will be considered in our future work. Furthermore, the coefficients of the precoder matrix can be optimized taking into account the effect of pilot contamination. Here, we expect to achieve a higher performance than that of the MMSE precoder, since the PE precoder offers more degrees of freedom for optimization. - Analysis of the impact of multiple antennas at the user terminals
Most works on massive MIMO assume single-antenna user terminals (UT). However, it would be interesting to investigate the improvement of the sum rate when several antennas are employed at the UTs. In particular, the rate gap between multiple-antenna UTs and single-antenna UTs could be investigated.
3. Physical-layer security
While traditionally data security in wireless communication systems was addressed only at the application layer using cryptographic methods, recently, there has been a paradigm shift and data security is already taken into account in the design of the physical (PHY) layer of communications systems [16]. In practice, PHY layer security can either serve as a complement to conventional security mechanisms or completely replace them, thereby avoiding complicated mechanisms and protocols for key distribution and management. For small-scale (conventional) MIMO systems, it has been shown that PHY layer security is facilitated by exploiting multiple transmit antennas and introducing artificial noise at the transmitter [17]. The combination of PHY layer security and massive MIMO is highly promising since massive MIMO systems offer more degrees of freedom and simpler transceiver designs compared to small-scale MIMO systems. While a large system PHY layer secrecy analysis of multi-user MIMO systems employing regularized channel inversion precoding has recently been reported in [18], a comprehensive analysis and design of PHY layer security mechanisms under the framework of massive MIMO systems is missing in the literature. For example, issues such as effective artificial noise generation and precoding for secure massive MIMO systems as well as the impact of pilot contamination on PHY security have not been addressed. Some of our recent results on this topic can be found in [19].
4. Transmission Design with Finite Alphabet Inputs
Most works on massive MIMO rely on the critical assumption of Gaussian input signals. Although Gaussian inputs are optimal in theory, they are rarely used in practice. Rather, practical communication signals usually are drawn from finite constellation sets, such as pulse amplitude modulation (PAM), phase shift keying (PSK) modulation, and quadrature amplitude modulation (QAM). These finite constellation sets differ significantly from the Gaussian idealization. Accordingly, transmission schemes designed based on the Gaussian input assumption may result in substantial performance losses when finite alphabet inputs are used for transmission in massive MIMO system. As a result, it is necessary to investigate the transmitter design for massive MIMO systems with finite alphabet inputs.
Performance of Precoder Design with Finite Alphabet Inputs
In Figures 3 and 4, the sum rate performance of a massive MIMO multiple access channel (MAC) with four users is depicted for different precoder designs and QPSK transmit symbols for the suburban and the urban scenarios of the spatial channel model (SCM) used in 3GPP standardization, respectively. We assume statistical CSI is available at the transmitter and consider the jointly-correlated fading MIMO channel. We employ the Gauss-Seidel algorithm together with stochastic programming to obtain the optimal covariance matrices of the users under the Gaussian input assumption. Then, we decompose the obtained optimal covariance matrices {Q1,Q2,⋯,QK}{Q1,Q2,⋯,QK} as Qk=UkΛkUHkQk=UkΛkUkH, and use Bk=UkΛ12kBk=UkΛk12, k=1,2,⋯,Kk=1,2,⋯,K as the precoders. Finally, we calculate the average sum rate for this precoding design for QPSK inputs. We denote the corresponding sum rate as „GP with QPSK inputs“. For the case without precoding , we set the precoders B1=B2=B3=B4=PNt−−√INtB1=B2=B3=B4=PNtINt. We denote the corresponding sum rate as „NP with QPSK inputs“. The sum rates achieved with the Gauss-Seidel algorithm and without precoding for Gaussian inputs are also plotted, and denoted as „GP with Gaussian input“ and „NP with Gaussian input“, respectively. We denote the proposed design for the MIMO MAC with finite alphabet inputs and statistical CSI as „FAP with QPSK inputs“. We observe from Figures 3 and 4 that, for QPSK inputs, the „FAP with QPSK inputs“ design achieves a better performance than the other precoding designs for both scenarios. For a sum rate of 2424 b/s/Hz, the SNR gains of the „FAP with QPSK inputs“ design over the „NP with QPSK inputs“ design for the suburban and the urban scenarios are about 4.54.5 dB and 3.53.5 dB, respectively. It is noted that the SNR gain for the suburban scenarios is larger than that for the urban scenarios. This is because the correlation of the transmit antennas is higher in suburban scenarios. Therefore, some subchannels are much stronger than the remaining subchannels. As a result, a proper precoding design will yield a larger performance gain. Also, the „GP with QPSK inputs“ design results in a substantial performance loss in both scenarios. This is because the Gauss-Seidel algorithm design implements a „water filling“ power allocation policy in this SNR region. As a result, when the SNR is smaller than a threshold, the precoders allocate most of the available power to the strongest subchannels and allocate little power to the weaker subchannels. Therefore, some eigenvalues of QkQk approach zero. For finite alphabet inputs, this power allocation policy may result in allocating most power to the subchannels that are close to saturation. This will lead to a waste of transmit power and impede the further improvement of the sum rate performance. This confirms that precoders designed under the ideal Gaussian input assumption may result in a considerable performance loss when adopted directly in practical systems with finite alphabet constraints and underlines the need for taking the finite alphabet input precoding into account for system design.

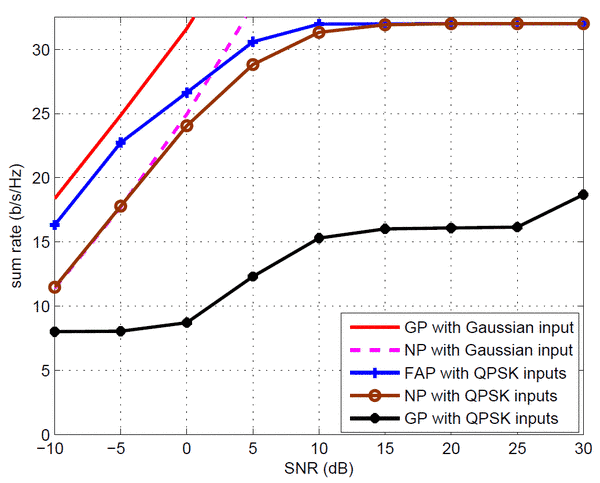
Research Challenges and Open Problems
There are many open research problems for transmission design in massive MIMO system with finite alphabet input:
- The precoder design for the massive MIMO MAC with finite alphabet inputs has been investigated only for some special cases. The general jointly-correlated Rician fading channel has not been considered yet. The impact of having a light-of-sight on the performance is unknown.
- The precoder design for the massive MIMO broadcast channel with finite alphabet inputs has been investigated only for the single-antenna user scenario. The extension to the multiple-antenna user scenario is needed.
- Security issues for massive MIMO systems with finite alphabet inputs has barely been investigated.
- The asymptotic mutual information for massive MIMO relay systems with finite alphabet inputs has been analyzed. However, a viable precoder design is still not known.
5. References
[1] J. Mietzner, R. Schober, L. Lampe, W. Gerstacker, and P. Hoeher, “Multiple-Antenna Techniques For Wireless Communications – a Comprehensive Literature Survey,” IEEE Communications Surveys and Tutorials, vol. 11, pp. 87-–105, Feb. 2009.
[2] G. Foschini and M. Gans, “On Limits of Wireless Communications in a Fading Environment when Using Multiple Antennas,” Wireless Personal Communications, vol. 6, pp. 311–335, Mar. 1998.
[3] E. Telatar, “Capacity of Multi-Antenna Gaussian Channels,” European Transactions on Telecommunications, vol. 10, pp. 585-–595, Feb. 1999.
[4] G. Caire and S. Shamai, “On the Achievable Throughput of a Multi-Antenna Gaussian Broadcast Channel,” IEEE Transactions on Information Theory, vol. 49, pp. 1691-–1706, July 2003.
[5] S. Vishwanath, N. Jindal, and A. Goldsmith, “Duality, Achievable Rates, and Sum-Rate Capacity of Gaussian MIMO Broadcast Channels,” IEEE Transactions on Information Theory, vol. 49, pp. 2658-–2668, Oct. 2003.
[6] D. Gesbert, M. Kountouris, R. Heath, C. Chae, and T. Salzer, “From Single User to Multiuser Communications: Shifting the MIMO Paradigm”, IEEE Signal Processing Magazine, vol. 24, pp. 36-–46, Sept. 2007.
[7] T. L. Marzetta, “Noncooperative Cellular Wireless with Unlimited Numbers of Base Station Antennas,” IEEE Transaction on Wireless Communications, vol. 9, no. 11, Nov. 2010.
[8] F. Rusek, D. Persson, B. K. Lau, E. G. Larsson, T. L. Marzetta, O. Edfors, and F. Tufvesson, “Scaling up MIMO: Opportunities and challenges with very large arrays,” IEEE Signal Processing Magazine, vol. 30, pp. 40–60, Jan. 2013.
[9] H. Q. Ngo, E.G. Larsson, T.L. Marzetta, “Energy and Spectral Efficiency of Very Large Multiuser MIMO Systems,” IEEE Transactions on Communications, vol. 61, no. 4, pp. 1436–1449, Apr. 2013.
[10] H. Ngo and E. Larsson, “EVD-Based Channel Estimation in Multicell Multiuser MIMO Systems with Very Large Antenna Arrays,” in Proc. International ITG Workshop on Smart Antennas, Kyoto, pp. 3249–-3252, Mar. 2012.
[11] R. Mueller, M. Vehkaperae, and L. Cottatellucci, “Blind Pilot Decontamination,” in Proc. International ITG Workshop on Smart Antennas, Stuttgart, Mar. 2013.
[12] H. Huh, G. Caire, H. Papadopoulos, and S. Ramprashad, “Achieving Massive MIMO Spectral Efficiency with a not-so-large Number of Antennas”, IEEE Transactions on Wireless Communications, vol. 11, pp. 3226-–3239, Sept. 2012.
[13] S. Wagner, R. Couillet, M. Debbah, and D. Slock, “Large System Analysis of Linear Precoding in Correlated MISO Broadcast Channels under Limited Feedback”, IEEE Transactions on Information Theory, vol. 58, pp. 4509–-4537, July 2012.
[14] C. S. et al., “Argos: Practical Many-Antenna Base Stations”, in ACM International Conference on Mobile Computing and Networking (MobiCom), pp. 1–-12, Aug. 2012.
[15] S.Zarei, W.Gerstacker, R.R. Muller, and R.Schober, “Low-complexity linear precoding for downlink large-scale MIMO systems”, in Proc. IEEE International Symposium on Personal, Indoor, and Mobile Radio Communications (PIMRC), 2013.
[16] Y. Liang, V. Poor, and S. Shamai, “Information Theoretic Security,” Foundations and Trends in Communications and Information Theory, vol. 5, pp. 355-–580, Apr. 2009.
[17] S. Goel and R. Negi, “Guaranteeing Secrecy Using Artificial Noise,” IEEE Transactions on Wireless Communications, vol. 7, pp. 2180-–2189, June 2008.
[18] G. Geraci, M. Egan, J. Yuan, A. Razi, and I. Collings, “Secrecy Sum-Rates for Multi-User MIMO Regularized Channel Inversion Precoding,” IEEE Transactions on Communications, pp. 3472-–3482, Nov. 2012.
[19] J. Zhu, R. Schober, and V. Bhargava, “Secure Transmission in Multi-Cell Massive MIMO Systems,” in Proc. IEEE Global Telecommunications Conference (Globecom) – Workshop on Trusted Communications with Physical Layer Security, 2013.
[20] C.-K. Wen and K.-K. Wong, “Asymptotic analysis of spatially correlated MIMO multiple-access channels with arbitrary signaling inputs for joint and separate decoding”, IEEE Transaction on Information Theory, vol. 53, pp. 252–268, Jan. 2007.
[21] C.-K. Wen, K.-K. Wong, and J.-C. Chen, “Asymptotic mutual information for Rician MIMO-MA channels with arbitrary inputs: A replica analysis”, IEEE Transaction on Communications, vol. 58, pp. 2782–2788, Oct. 2010.
[22] B. M. Zaidel, R. R. Mueller, A. L. Moustakas, and R. Miguel, “Vector precoding for Gaussian MIMO broadcast channels: Impact of replica symmetry breaking”, IEEE Transaction on Information Theory, vol. 58, pp. 1413-1440, Mar. 2012.
[23] M. A. Girnyk, M. Vehkapera, and L. K. Rasmussen, “Large-system analysis of correlated MIMO multiple access channels with arbitrary signaling in the presence of interference,” online available: arxiv.org/abs/1305.4755v1.
[24] M. A. Girnyk, M. Vehkapera, and L. K. Rasmussen, “Large-system analysis of the KK-hop AF MIMO relay channel with arbitrary inputs”, IEEE International Symposium on Information Theory, Istanbul, Turkey, Jul. 2013.
[25] Y. Wu, C.-K. Wen, C. Xiao, X. Gao, and R. Schober, “Linear MIMO precoding in jointly-correlated fading multiple access channels with finite alphabet signaling”, online available: arxiv.org/abs/1401.5401.